Unveiling anomalies for safer connections
Innovative project insights from the Cyber Valley Research Fund
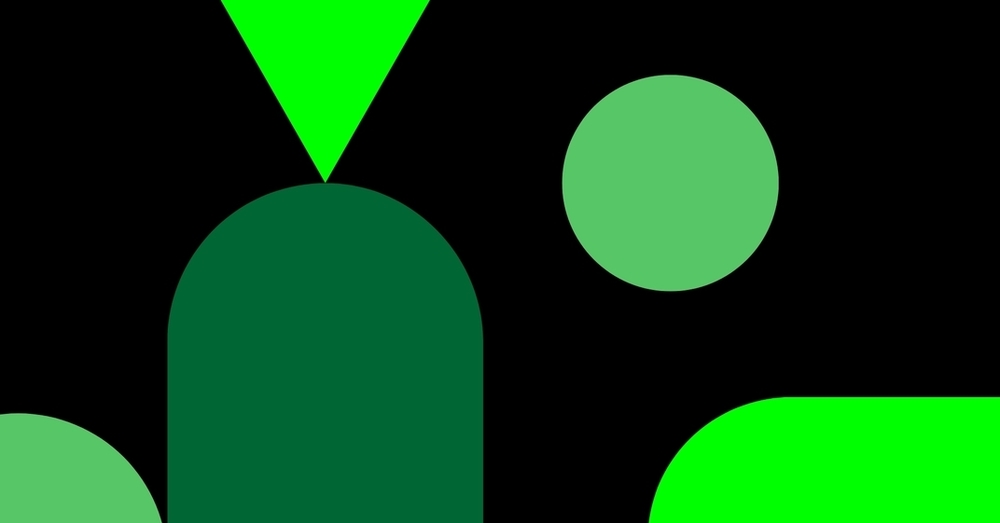
A recently concluded research project funded by the Cyber Valley Research Fund has highlighted the critical significance of identifying abnormal behavior within social networks and beyond. Led by Dr. Caterina De Bacco, Research Group Leader, alongside her team members Hadiseh Safdari, Postdoctoral Researcher, and Martina Contisciani, Ph.D. Student, the project "Anomaly Detection in Networks: Reciprocated and Dynamic Networks," has been a pivotal endeavor. It was supported by the Cyber Valley Research Fund since September 2021 and concluded in December 2023.
Six partners within the Cyber Valley Community have generously contributed to the Cyber Valley Research Fund, totalling five million euros, to facilitate independent basic research. Research groups were invited to submit project proposals for the Research Fund, which the Cyber Valley Public Advisory Board evaluated, taking into account scientific excellence, as well as ethical and societal considerations.
The research project's core objective was anomaly detection within networked datasets, primarily focusing on identifying abnormal behavior across diverse network types, including social, communication, and transportation networks. The project thereby aimed to provide early warnings of potential threats or irregularities.
The research introduced a novel approach that combined community structure with anomaly detection, enabling the identification of anomalies as edges that deviate from regular patterns. This innovative technique leveraged the structure of dynamic networks over time and inferred anomalies by integrating domain knowledge, such as community membership, into the analysis.
The project yielded promising results, showcasing the model's accuracy in capturing both community and anomaly structures, and its significant performance in predicting links within networks. The developed ideas and methodologies hold pertinent applications, particularly in fields such as cybersecurity and social network analysis, offering promising avenues for addressing critical challenges like fraud detection and network security enhancement. This includes applications such as uncovering fake users on social media platforms and detecting fraudulent activities in financial transactions.
Furthermore, the research provided valuable insights into the dynamics of real-world networks by leveraging community structure alongside dynamic patterns of edge additions and deletions. Ultimately, the project not only advanced scientific understanding of network dynamics but also holds substantial potential for meaningful real-world impact. These discoveries herald new possibilities for addressing complex issues and enhancing the security and efficiency of various network systems.
This project has yielded three peer reviewed publications:
- “Anomaly detection and community detection in networks”, H. Safdari and C. De Bacco, J Big Data 9, 122, https://doi.org/10.1186/s40537-022-00669-1, 2022.
- “Anomaly, reciprocity, and community detection in networks”, H. Safdari, M. Contisciani and C. De Bacco, Phys. Rev. Research 5, 033084, https://link.aps.org/doi/10.1103/PhysRevResearch.5.033084, 2023.
- “Community detection and anomaly prediction in dynamic networks”, H. Safdari and C. De Bacco, work in progress, 2024. Paper and preprint expected to be submitted by April 2024.
It also yielded two inventions:
Related Articles
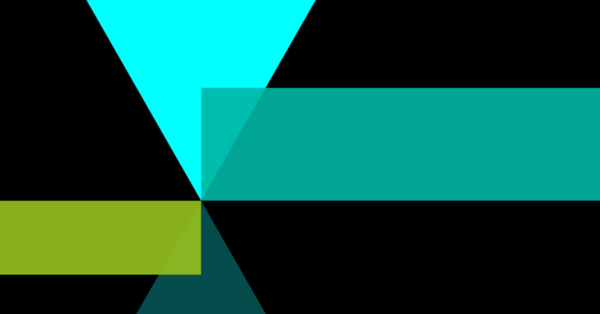
Cyber Valley Research Fund project optimizes focus and pr...

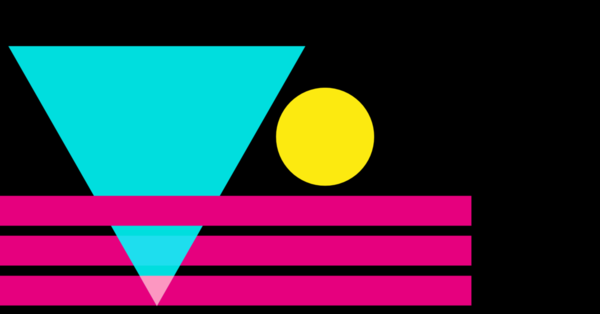
Cyber Valley Research Fund drives advancements in soft ro...

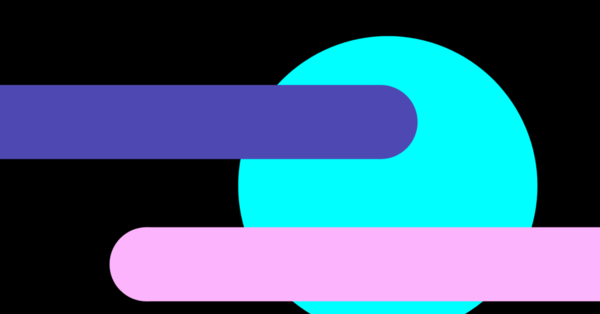
Cyber Valley Research Fund improves human decision-making...
